SLF, Swiss Institute for Snow & Avalanche Research Develops AI – Avalanche Intelligence
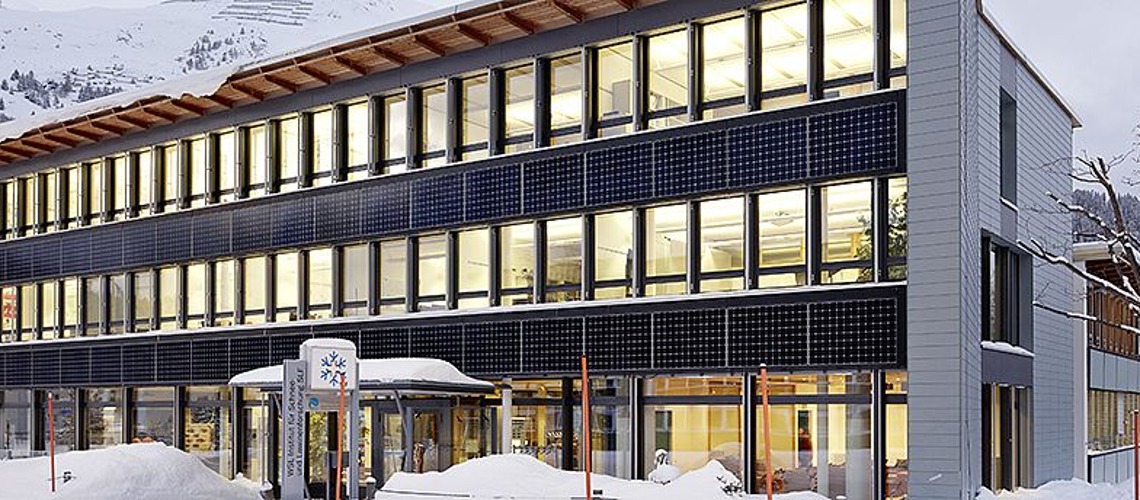
Machine-trained algorithms estimate the current avalanche situation just as well as humans – with different approaches, strengths and weaknesses.
Forecast for Saturday, February 10, 2024, for southern Switzerland was issued using a forecast model developed with a lot of data and the machine learning (ML) method: avalanche warning level 3 (major) with a trend towards 4 (major).
After a three-year test phase, this year a model will be at the table for the first time, figuratively speaking, when the avalanche warning service employees decide which region they should assign which danger level to. The three-year lead time has already shown that the model's predictions are often good. “Sometimes they are clearly not, but we are also wrong sometimes,” says avalanche warner Frank Techel. On February 10th, the machine is of much the same opinion as its three human colleagues (see picture).
The computer has learned to interpret the in-house physical model for simulating the SNOWPACK snowpack, some of which the avalanche warning service has been using for decades. In other words: What is new is not the use of algorithms in avalanche warning, what is new is that algorithms analyze and evaluate the results of other models such as the simulation of the snow cover and independently assess the situation.
The project started in 2019 and is based on an initiative by SLF leader Jürg Schweizer. A team of SLF researchers and avalanche warning officers worked on this, together with colleagues from the Swiss Data Science Center. For two years, physicist Cristina Pérez carried out experiments with different methods and data sets, processed data and ultimately trained the model with it. She used weather data and snow cover simulations from twenty years, which are based on measurements from the intercantonal measurement and information system IMIS. This approach is called machine learning. On the one hand, the challenges included choosing the parameters so that the algorithms became more and more precise. “On the other hand, it was difficult to obtain good accuracy for avalanche warning level four, since this high warning level had only occurred rarely in twenty years, so the database was quite small,” says Pérez. The employees of the avalanche warning service call the platform on which they view the various ML models Palantir, after the seven crystal balls from JRR Tolkien's fantasy world Arda with the most famous continent of Middle-earth, which show scenes far away in space and time.
The human employees of the avalanche warning service use the same data and models for their work as the computer. But they also use information such as current observations and feedback from the field. This data is not available to the computer. The algorithm only uses simulations of the snow cover as input. On the other hand, for reasons of time, people select the data that is relevant to them from the amount of data; the machine does not select. “Models allow a spatial and temporal resolution that we humans will never achieve,” explains Techel. Humans and machines complement each other. The algorithms help interpret basic data sets. Both sides also make mistakes. “The good thing is that the models make different mistakes than we do,” says Techel. This gives the avalanche warning service a second, independent opinion and can reconsider its current result for the avalanche bulletin if there are major discrepancies.
The team is currently developing the project further and wants to better combine human and machine predictions in the future. “This also includes a more intuitive presentation of the results for the avalanche warning service,” says Techel.
https://www.slf.ch/de/